Bin Yu to deliver Hotelling Lectures
April 13, 2022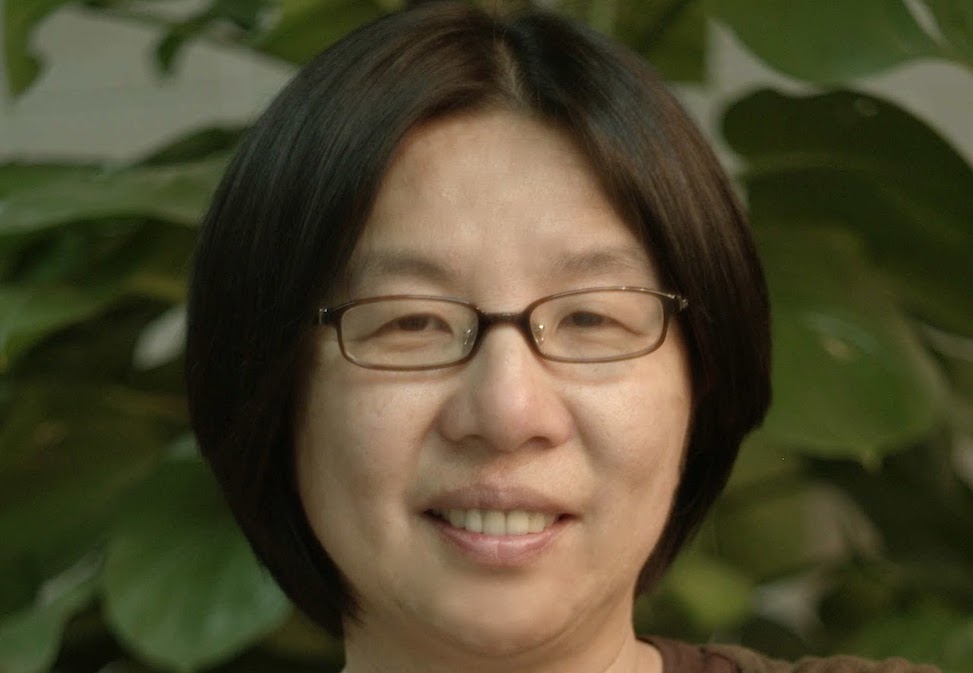
The Hotelling Lectures are an annual event in the Department of Statistics & Operations Research at the University of North Carolina – Chapel Hill, honoring the memory of Professor Harold Hotelling our first chairman. This year we are honored to have Professor Bin Yu from the University of California at Berkeley deliver our two Hotelling lectures which are open to the public.
Biography
Bin Yu is Chancellor’s Distinguished Professor and Class of 1936 Second Chair in the departments of statistics and EECS at UC Berkeley. She leads the Yu Group which consists of students and postdocs from Statistics and EECS. She was formally trained as a statistician, but her research extends beyond the realm of statistics. Together with her group, her work has leveraged new computational developments to solve important scientific problems by combining novel statistical machine learning approaches with the domain expertise of her many collaborators in neuroscience, genomics and precision medicine. She and her team develop relevant theory to understand random forests and deep learning for insight into and guidance for practice.
She is a member of the U.S. National Academy of Sciences and of the American Academy of Arts and Sciences. She is Past President of the Institute of Mathematical Statistics (IMS), Guggenheim Fellow, Tukey Memorial Lecturer of the Bernoulli Society, Rietz Lecturer of IMS, and a COPSS E. L. Scott prize winner. She holds an Honorary Doctorate from The University of Lausanne (UNIL), Faculty of Business and Economics, in Switzerland. She has recently served on the inaugural scientific advisory committee of the UK Turing Institute for Data Science and AI, and is serving on the editorial board of Proceedings of National Academy of Sciences (PNAS).
Veridical Data Science: the practice of responsible data analysis and decision-making
Tuesday, April 19, 2022 (4:00-5:00pm 209 Manning Hall)
Reception following the lecture 5:00-6:00pm in the 3rd Floor lounge of Hanes Hall
“A.I. is like nuclear energy — both promising and dangerous” — Bill Gates, 2019.
Data Science is a pillar of A.I. and has driven most of recent cutting-edge discoveries in biomedical research and beyond. In practice, Data Science has a life cycle (DSLC) that includes problem formulation, data collection, data cleaning, modeling, result interpretation and the drawing of conclusions. Human judgement calls are ubiquitous at every step of this process, e.g., in choosing data cleaning methods, predictive algorithms and data perturbations. Such judgment calls are often responsible for the “dangers” of A.I. To maximally mitigate these dangers, we developed a framework based on three core principles: Predictability, Computability and Stability (PCS). Through a workflow and documentation (in R Markdown or Jupyter Notebook) that allows one to manage the whole DSLC, the PCS framework unifies, streamlines and expands on the best practices of machine learning and statistics – taking a step forward towards veridical Data Science. In this lecture, we will illustrate the PCS framework through the development of of iterative random forests (iRF) for predictive and stable non-linear interaction discovery and through using iRF and UK biobank data to find gene-gene interactions driving, respectively, red-hair and a heart disease called hypertrophic cariomyopathy.
Interpreting deep neural networks towards trustworthiness
Wednesday, April 20, 2022 (3:30-4:30pm 120 Hanes Hall)
Reception prior to the lecture 3:00-3:30pm in the 3rd Floor lounge of Hanes Hall
Recent deep learning models have achieved impressive predictive performance by learning complex functions of many variables, often at the cost of interpretability. This lecture first defines interpretable machine learning in general and introduces the agglomerative contextual decomposition (ACD) method to interpret neural networks. Extending ACD to the scientifically meaningful frequency domain, an adaptive wavelet distillation (AWD) interpretation method is developed. AWD is shown to be both outperforming deep neural networks and interpretable in two prediction problems from cosmology and cell biology. Finally, a quality-controlled data science life cycle is advocated for building any model for trustworthy interpretation and introduce a Predictability Computability Stability (PCS) framework for such a data science life cycle.